18 Nov Introduction to Conversion Rate Optimization (CRO)
Introduction to Conversion Rate Optimization (CRO)
Over 100 years ago, businessman John Wanamaker famously said, “Half the money I spend on advertising is wasted. The trouble is, I don’t know which half!”
That question has plagued marketers since the dawn of time, but lucky for you, some very smart techies have figured out how to answer it—at least when it comes to web-based marketing and advertising.
In this post about Conversion Rate Optimization (CRO), you’ll learn:
What is Conversion Rate Optimization?
A “conversion” is digital-marketing-speak to describe a customer or prospect performing a desired action. That includes things like:
- Opening an email
- Clicking on an ad
- Subscribing to a blog
- Scheduling an appointment for a product demo
- Purchasing a product
Conversion Rate Optimization is all about increasing the ratio of people who perform these desired actions. CRO involves making informed decisions about testing different website elements to see what produces the best results. In other words, CRO does away with the guesswork, so unlike Mr. Wanamaker, you’ll never have to wonder what works and what doesn’t. In fact, you’ll continually refine your content to produce better and better results.
We’ll get into how to run those tests below (using techniques called A/B testing and multivariate testing), but first things first! Before you start testing, you’ll need to identify which website elements you want to optimize.
What elements should you test?
You can test all kinds of things, including:
- Headlines
- Calls-to-action
- Email subject lines
- Website graphics and images
- Forms
- Ad copy
- And more
However—you don’t just want to start randomly testing everything you can think to test. That’s not how effective CRO works. Getting the greatest ROI out of your A/B tests means testing elements that, when changed, are most likely to increase conversions.
The best way to identify those elements is by studying user behavior on your website and gathering visitor feedback. Here are a few tools you can use to gather data and make intelligent decisions about which elements you want to test.
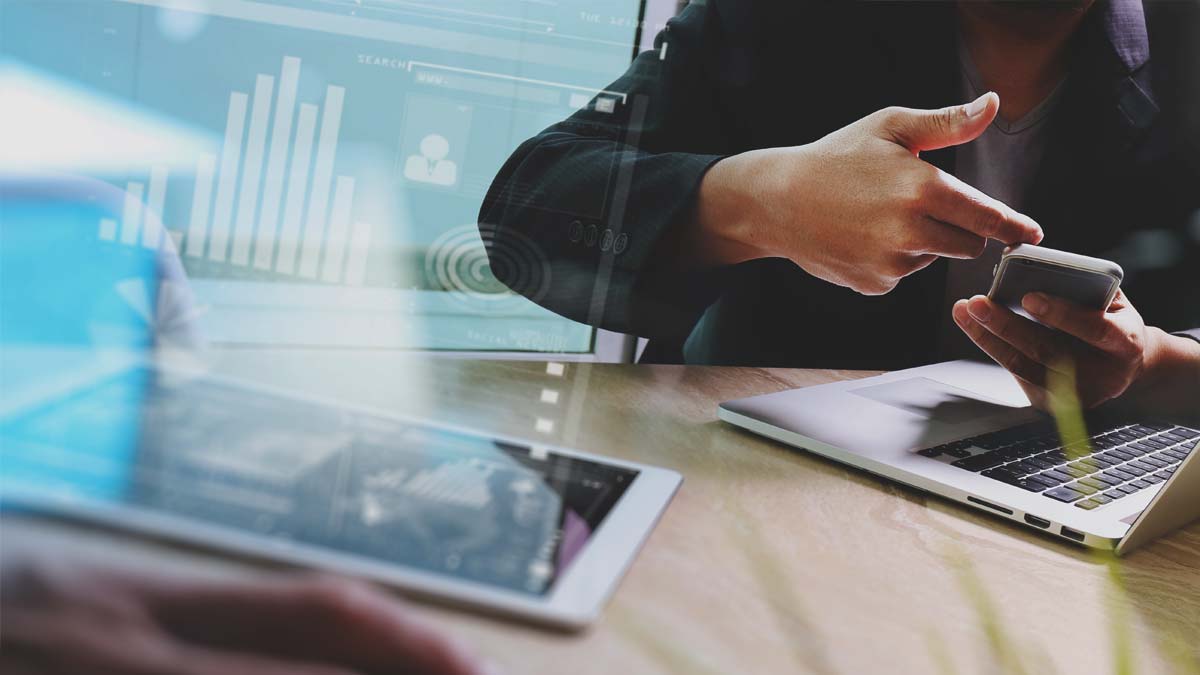
Google Analytics
Google analytics will show you where your web traffic comes from, which pages people visit, and where they drop off your website. This can help you figure out where to focus your CRO efforts.
Example: After studying Google analytics, you learn that most of your visitors land on your Home page, then they visit your Product page, then they go to your Pricing page—and that’s where most of them drop off and fail to return. Those who stick around and visit pages beyond the Pricing page tend to become paying customers, so you determine that your Pricing page is the weakest link in your sales funnel. That’s where you’ll focus your attention for now.
Heatmaps
Heatmaps show where people click, hover, and scroll on your page (in aggregate). On a heatmap that measures clicks (i.e., a click-map), the areas getting the most clicks will appear bright red, and the areas with less attention appear in colder colors (yellows, greens, and blues). On heatmaps that measure scrolling, you can see how far people read (i.e., as you move down the page, the scroll-map will transition from red to blue).
Example: You’re concerned that not enough people are willing to sign up for a free consultation, so you look at a heatmap of your sign-up form. You discover that the majority of people stop at the phone number field and don’t scroll any farther down the page, so you hypothesize that removing the phone number field (or making it an optional entry) could lead to more signups (i.e., conversions).
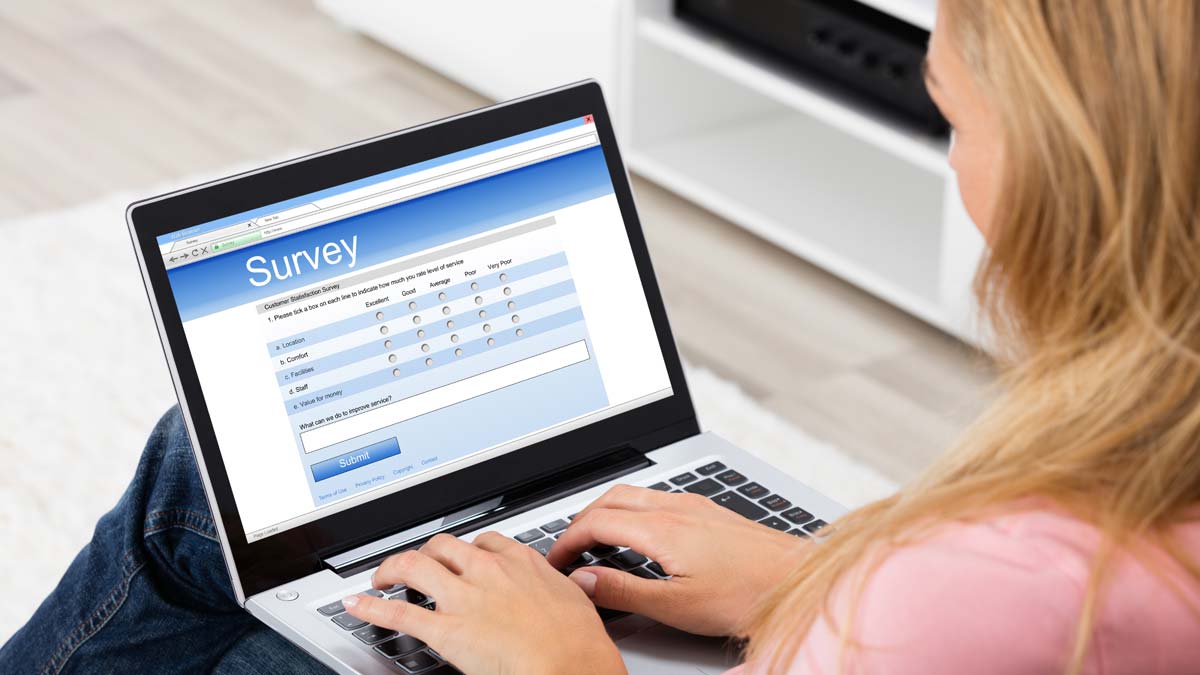
Surveys
On-page surveys slide up from the bottom of the screen and ask the user questions about what they want from the website, whether they’ve found what they’re looking for, etc. You can ask anything in these surveys, but if you’re new to surveying your customers, consider asking open-ended questions. Open-ended questions (as opposed to multiple-choice questions) will alert you to possible barriers to conversion that you never knew existed.
Example: Users visit your pricing page, but they leave without moving further along in your sales funnel. Your CEO is worried that the pricing is too high, but rather than jumping to conclusions and taking revenue off the table, you run a survey asking, “How can we improve this page?”
Your users might tell you that the pricing is too high, but maybe not? They could tell you that the pricing is simply confusing (that can be a big problem for many subscription-based businesses). If that’s the case, you can tweak your pricing models and test out new ones to see if that makes a difference.
Additional ways to understand your visitors
User session recordings: Sessions recording tools allow you to play back recordings of individual making their way through your website. Even though these are anecdotes rather than data (since you’re only observing one person at a time), it’s still a good practice to see how actual users respond to your content.
Customer interviews: Sitting down and speaking with your customers can build empathy and help you stumble across customer needs and drives you would’ve never considered. Again, this is anecdotal information (as opposed to data), but at this stage you’re looking for ideas to explore. Like a scientist, you’re looking to generate hypotheses that you will put to the test in the next step: A/B testing.
A/B Testing
Once you know what you want to study, you can use a testing platform like Optimizely or AB Tasty to drive equal amounts of traffic to two different versions of your website.
Example: A wedding planning firm comes up with two headlines for a landing page—one is creative and the other is direct and to-the-point.
Out of 500 visitors, 250 go to version A and 250 go to version B. Version A converts 2 visitors (conversion rate = 0.8%) and Version B converts 7 visitors (conversion rate = 2.8%).
There’s a clear winner, right? Not so fast… first you have to figure out whether your results are statistically significant.
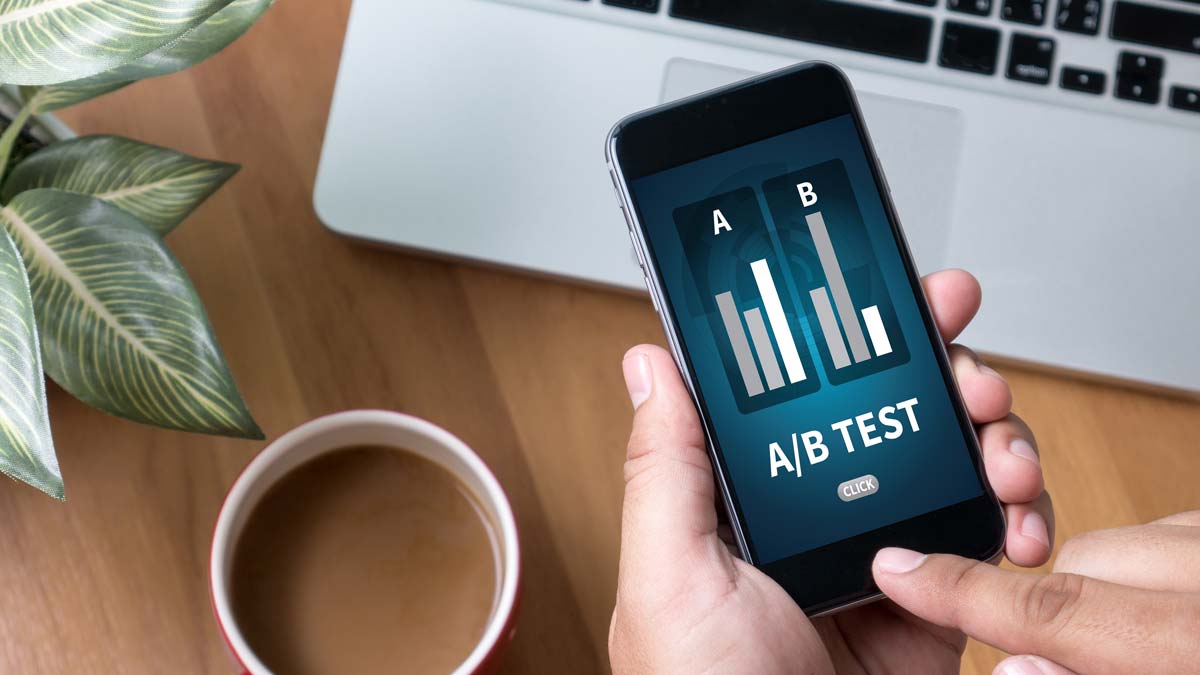
What is statistical significance?
Statistical significance calculates the odds that your results didn’t occur by random chance.
For example, imagine if you went to U.C. Berkeley in California and asked three students who they planned to vote for in the 2020 U.S. presidential election. If two out of three said they planned to vote for Donald Trump, you’d be wrong to conclude that Donald Trump was more popular than his Democratic rival among U.C. Berkeley students. These results could have happened by random chance, and you’d need a larger sample size to be more confident in your assessment.
You can use a statistical significance calculator to determine your desired level of statistical significance in an A/B test (rather than using a long, drawn-out manual formula). What’s important to know is that your statistical significance will increase or decrease based on two factors:
- Sample size
- The difference between Version A’s performance and Version B’s performance
In other words, if Version B outperforms Version A (or vice versa) by a significant margin, you can get away with a smaller sample size. On the other hand, if conversion rates are close, you need a much larger sample size to show that the difference probably wasn’t caused by random variation.
In our example, Version B converted visitors at a rate 3.5 times that of Version A. When you plug those numbers into the calculator, you’ll get a statistical significance of 96%, meaning there’s a 96% chance that Variation B didn’t win by chance.
In other words, Variation B really is the winner in this case… and you can be 96% sure of it.
What level of statistical significance do you need? That varies based on the level of risk you’re willing to accept, but a common standard in CRO is 95%. You can lower your desired level of statistical significance if you’re willing to accept greater risk.
What if you don’t get much traffic? A/B testing requires a certain amount of traffic to give you statistically significant results. If you don’t get much traffic, you’ll have to be very judicious about what you test since it will take a lot of time to get statically significant data.
What is multivariate testing?
Multivariate testing involves testing more than one element at the same time.
For example, if you wanted to test three different headlines and two different images at the same time, your testing software will create six different versions and run equal amounts of traffic to all of them (3 headlines x 2 images = 6 versions).
Advantage of multivariate testing: It’s more efficient since you can test everything at the same time.
Disadvantage of multivariate testing: It requires much more traffic to get statistically significant results.